Whether they know it or not, all sports fans, even casual ones, have probably encountered data analytics, from Sportsnet graphics to a player’s batting average or save percentage. The sheer amount of data used in sports analysis can be intimidating for someone unfamiliar with analytics, but gaining a basic understanding of this data and how they factor into games is easy to learn.
Analytics is the collection and use of statistical data to help teams and individuals measure their performance and improve upon it. These statistics can be basic, like a basketball player’s rebounds, or more advanced, like a football team’s intended air yards per pass attempt. Three major types of analytics are often used simultaneously: Descriptive analytics gather information about previous games, predictive analytics predict outcomes of future competitions, and prescriptive analytics recommend how to improve performance.
Although the use of analytics is now standard across most sports leagues, the practice has only recently become mainstream. Billy Beane, the General Manager of Major League Baseball’s Oakland Athletics from 1998 to 2016, is believed to be the first sports executive to build their team’s lineup and strategy based on data analytics. The Athletics’ successful 2002 season, which culminated in a West Division title, was chronicled in the 2003 book and the 2011 movie Moneyball.
In the two decades since, many other sports have started to use analytics to monitor player data and a wealth of information has been available to the public. Dr. Timothy Chan, professor in the Department of Mechanical and Industrial Engineering at the University of Toronto, has followed the increased use of analytics in hockey for years.
“Back in 2009, when we were starting our hockey work, the data and the statistics that were being captured were still very simple,” Chan said in an interview with The McGill Tribune. “In the 10 years since, there has been an explosion of data that leads to advanced stats that we can calculate. The interest [has grown] from pro all the way down to amateur and other levels of sport.”
The applications of analytics in professional sports are endless: Teams use prescriptive models to call plays and create player profiles to decide who to sign or draft. These profiles are often used in contract negotiations and when forming game lineups.
Despite the benefits of analytics, they are not without their troubles, as sports are by and large a “boys’ club,” and analytics is no exception. An estimated 85 per cent of attendees of the 2015 MIT Sloan Sports Analytics Conference were male, and only 22 out of 153 speakers were women. Chan is currently working on a project that aims to provide empirical data on the demographic makeup of the sports analytics community.
“We are trying to get a baseline of what is the state of diversity and inclusion in the field,” Chan said.
According to Stephen Gill, BCom ‘20, founder of the football analytics blog twitrsports, and master’s student at Northwestern University’s Analytics graduate program, the inaccessibility of data can also be a barrier to understanding them.
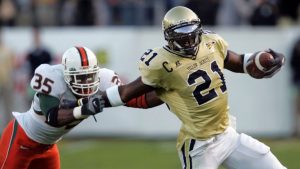
“There is a pretty big knowledge gap between the practitioners and the audience,” Gill said. “The practitioners don’t try as much as they should to make [information] accessible, and the audience sometimes can be resistant to change.”
McGill Athletics has started to use analytics for some of its varsity teams, including the men’s ice hockey team. Gianfranco Giuliano, the Director of Analytics for the Redbirds Hockey team, uses analytical data to strategize for games. Their statistical database contains over 75,000 data points, ranging from information about zone entries and exits, to expected goals, and passing networks. Giuliano said he creates post-game reports by analyzing video footage from games and then provides coaches with the data.
“The most important part of my job is communication,” Giuliano said. “Being able to relay these concepts in some form of a digestible, actionable kind of way is the most imperative. [You want to] give [coaches] something they can implement, whether it be a strategy, a lineup change, a concept, […] something where they can find some kind of a positive impact.”
In addition to game reports, Giuliano creates player profiles to better understand how players perform together on the ice.
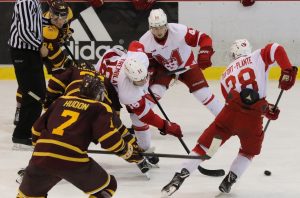
“Once you have enough of those profiles amassed, 12 forwards and six [defence], then you can figure out why guys should be playing together, why they should not be playing together, and what works and what does not work,” Giuliano said.
After delving into sports analytics, finding a foothold to produce and share one’s work can be daunting. Some specific knowledge needed—expertise in math and programming languages—and where to find information is not made immediately clear. While one can understand descriptive graphics online without deep knowledge of math and programming, specific skills are needed to produce original analytics data.
A few key steps will go a long way toward appreciating analytics, but the most important is understanding the sport. Reading about Jonathan Drouin’s offensive zone deployment is not without knowing the difference between a power play and penalty kill.
The next step, Gill recommends, is to read as many books, blogs, or Tweets as possible.
“There are a lot of people who have written stuff in the past about every sport,” Gill said. “Twitter is a good spot to get a feel for the lay of the land, and from there, you can tell that there are [websites] that specialize in specific sports.”
Creating original analytics work, however, does require specific coding skills that can only be developed with time and effort.
“Entry-level analysts really need […] good programming skills, so probably Python or R, and a good grasp of basic statistics,” Chan said.
While a formal education in statistics or computer science is helpful, many of the skills needed for analysis can be learned through websites like Coursera, Khan Academy, and Harvard Online Courses that offer free classes on statistics, programming, and data science. For those less mathematically-inclined, however, Giuliano recommends exploring data visualization tools like Tableau or PowerBI—programs that generate interactive graphics and reports—as well as Excel.
Chan, Gill, and Giuliano all emphasize that the most crucial step to embarking on an analytics journey is to begin proactive learning. The process starts by thinking of a question and finding data to provide an answer. Next, publicize the data in any way possible by starting a blog, using Twitter, or attending conferences, and talking to people in the sports analytics community. When it comes to becoming involved in analytics, Giuliano stressed the importance of asking for opportunities.
“Honestly, just ask,” Giuliano said. “It sounds so naive and utopian just to say it out loud, but […] people will always appreciate the help.”
Innovations in analytics are made every day, and conferences and contests like the NFL Big Data Bowl and the MIT Sloan Sports Analytics Conference encourage new ideas. As the world of analytics evolves, there are several new opportunities to learn and collaborate. With a clear picture of the tools available and how to access them, there is nothing stopping anyone from giving analytics a try.